A machine learning algorithm for detecting ripeness levels in papaya fruit could help both shoppers and producers.
If you’re in the market to buy fresh papayas, it can be a challenge to figure out ripeness based on peel color without also squeezing the fruit to test for softness. A Brazilian research group could make life easier for both shoppers and producers in the near future with a computer vision algorithm that estimates ripeness based on images alone.
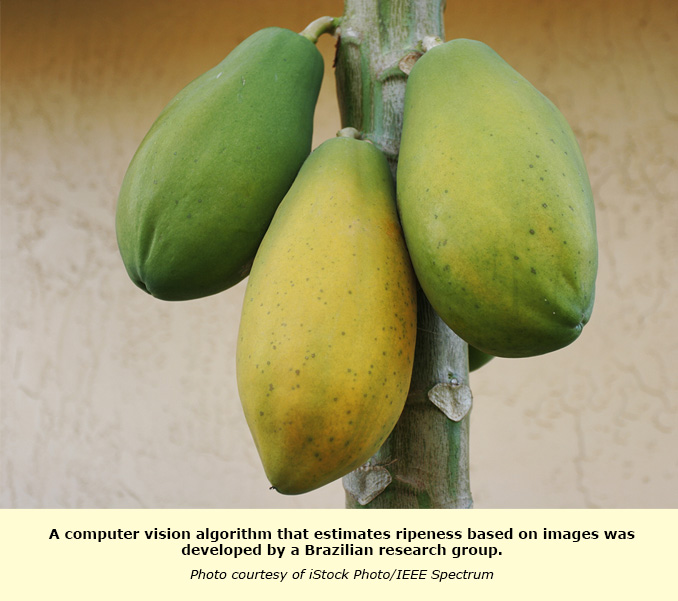
Last year, the United States alone imported more than US $107 million worth of fresh papayas as the world’s largest papaya import market. The computer vision software could enable papaya growers to maximize the value of their fruit by sending the ripest papayas to local markets and saving less ripe papayas for export, says Douglas Fernandes Barbin, a researcher in the department of food engineering at the University of Campinas in São Paulo, Brazil. But he and his colleagues also want to help individual shoppers get their money’s worth in grocery aisles.
“The idea is to later develop an app for the consumer,” Barbin says. “The consumer could go to the market and take a picture and find the mature stage and decide which one to buy to eat that day or over the next few days.”
The University of Campinas researchers teamed up with computer scientists from Londrina State University in Londrina, Brazil to develop the machine learning approach that achieved an overall ripeness detection accuracy of 94.7 percent. Their work appears in the February issue of the journal Computers and Electronics in Agriculture.
Measuring ripeness—and identifying relevant features for ripeness—was one of the biggest challenges. The researchers started out with a government guidance chart that listed five levels of papaya ripeness. But they soon consolidated ripeness levels into three maturity levels based on visual inspection: Visually, the outer peel of the golden papayas starts out green and yellows as the fruit ripens. They further verified the three levels with additional testing based on each fruit’s pulp firmness.
Training the machine learning algorithm also proved an unexpected challenge: It required a diverse selection of papayas. Researchers had hoped to get a large number of papayas from a local producer but eventually found themselves buying 57 golden papayas at a local market in Campinas.
Both the hardware and software components of the project proved relatively straightforward. On the hardware side, researchers built a boxy contraption with a consumer digital camera and light bulbs positioned on the ceiling to take illuminated pictures of the papaya samples. Success with such consumer-grade technology means this approach could be adapted fairly readily to commercial applications.
On the software side, the researchers considered a number of different machine learning algorithms before settling upon the common random forest classifier. This approach enabled the researchers to clearly see how different papaya features factored into the machine learning algorithm’s results. “We could see which features are really providing useful information about the fruit,” Barbin explains.
A deep learning approach based on neural networks also might have yielded good results for visually identifying ripe papayas. But the Londrina State University colleagues were wary of the black box nature of deep learning algorithms that usually makes it extremely difficult to figure out how deep learning comes up with any given result. Furthermore, a deep learning approach would have required a potentially far greater sample of papayas in the training dataset to achieve reasonable accuracy.
Despite the overall 94.7 percent accuracy, the machine learning accuracy in identifying the appropriate level of ripeness fell to just 84.4 percent for fruit samples in the third stage of maturity. This comes in part from the fact that the peel color does not correspond perfectly with the inner state of the papaya’s pulp firmness. Still, the researchers anticipate improving upon this performance with a wider variety of samples in the future.
Such computer vision software could prove a handy guide for ordinary shoppers if the research team can secure the necessary funding to develop a consumer-facing smartphone app. But on the business end, it could find its way into the hands of papaya producers even sooner. The computer vision approach would also potentially lay the groundwork for automated harvesting by agricultural robots farther down the road.
“Papaya is very important for the Brazilian economy, especially the northeastern side of the country, ” Barbin says. “If a producer can really decide if it’s fully ripe and ready for harvest or processing, that can reduce food waste and add value to the product.”
Written by Jeremy Hsu, IEEE Spectrum